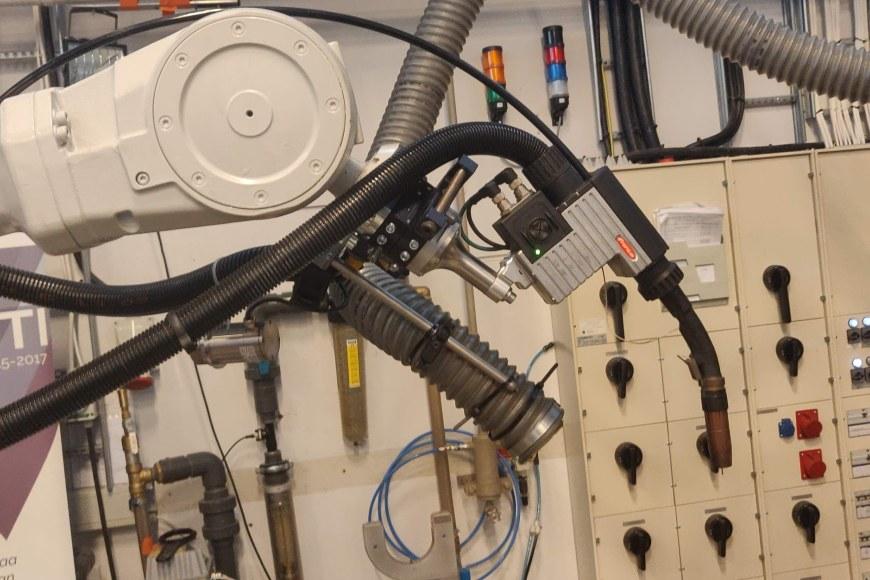
Our research group is composed of skilled professionals and researchers with profound knowledge in manufacturing technologies and engineering design. The group is combining modelling, simulation and experimental efforts in the field of manufacturing systems. The specificity of the group is a unique focus on metamodeling approaches for engineering simulations and decision support. The context is specifically the development of robust metamodeling approaches in scarce data context.
Research focus and goals
The group research focus is the following:
- Modeling and simulation of manufacturing systems,
- Early integration of manufacturing considerations in the system development process.
- Efficient dynamic control and development of additive manufacturing methods based on Directed Energy Deposition,
- Knowledge encoding in the form of causal graphs,
- Integration of existing knowledge and design of artificial neural networks topologies using causal graphs,
- Dimensional analysis theory and its combination with causal graphs,
- Causal graph networks for decision-making support,
- Multi-Disciplinary Optimization and creativity support for Engineering,
- The early design process, automatic extraction, and analysis of requirements,
- Metrics for system design and manufacturing,
- Machine learning with Bayesian Networks using Causal graphs as a precursor.
The team is aiming for three complementary targets. The main outcome of the research is a multi-purpose colored causal graph approach supporting the following goals.
The first goal is to create, for engineering design and manufacturing disciplines, a new form of integrative metamodeling approaches supporting the integration of multiple models coming from various disciplines and developed via heterogeneous modeling and simulation approaches.
The second goal is to provide a system analysis method for ill-defined problems requiring solely qualitative information.
The third goal is to use the causal graphs as topology design support and precursors for different machine learning methods. They serve as well as pre-training method.
Impact
The research, developed in our group, the intention is to transform the early design, manufacturing and optimization disciplines by providing an automation layer for efficient decision support, modelling, simulation and control in a scarce data environment.
The result can be new forms of modeling, simulation and control tools for engineering disciplines.
Following are list of publications from the research group:
Sr. | Conference Papers | Link |
---|---|---|
1 | Improving worker health and safety in wire arc additive manufacturing: A graph-based approach. | https://doi.org/10.1016/j.procir.2020.01.116 |
2 | A dimension reduction method for efficient optimization of manufacturing performance | https://doi.org/10.1016/j.promfg.2020.01.070 |
3 | Probabilistic modeling of defects in additive manufacturing: a case study in powder bed fusion technology | https://doi.org/10.1016/j.procir.2019.03.234 |
4 | Tracing the interrelationship between key performance indicators and production cost using Bayesian Networks | https://doi.org/10.1016/j.procir.2019.03.136 |
5 | Knowledge-based artificial neural network (KB-ANN) in engineering: Associating functional architecture modeling, dimensional analysis and causal graphs to produce optimized topologies for KB-ANNs | https://doi.org/10.1115/DETC2018-85895 |
6 | Dimensional analysis conceptual modeling supporting adaptable reasoning in simulation-based training | https://doi.org/10.1109/SYSOSE.2018.8428785 |
7 | Dimension reduction and decomposition using causal graph and qualitative analysis for aircraft concept design optimization | https://doi.org/10.1115/DETC2017-67601 |
Sr. | Journal Articles | Link |
---|---|---|
1 | Knowledge production, sharing, and design in an age of fundamental transformations | https://doi.org/10.3233/JID200010 |
2 | Systematic manufacturability evaluation using dimensionless metrics and singular value decomposition: a case study for additive manufacturing | https://doi.org/10.1007/s00170-020-06158-0 |
3 | Graph-based metamodeling for characterizing cold metal transfer process performance. | https://doi.org/10.1520/SSMS20190026 |
4 | Knowledge-based design of artificial neural network topology for additive manufacturing process modeling: A new approach and case study for fused deposition modeling | https://doi.org/10.1115/1.4042084 |
5 | A conceptual design and modeling framework for integrated additive manufacturing | https://doi.org/10.1115/1.4040163 |
6 | Function modeling combined with physics-based reasoning for assessing design options and supporting innovative ideation | https://doi.org/10.1017/S0890060417000403 |
7 | Comparative environmental impacts of additive and subtractive manufacturing technologies | https://doi.org/10.1016/j.cirp.2016.04.036 |
Leader
Eric Coatanea
Professor, Design & Manufacturing technologies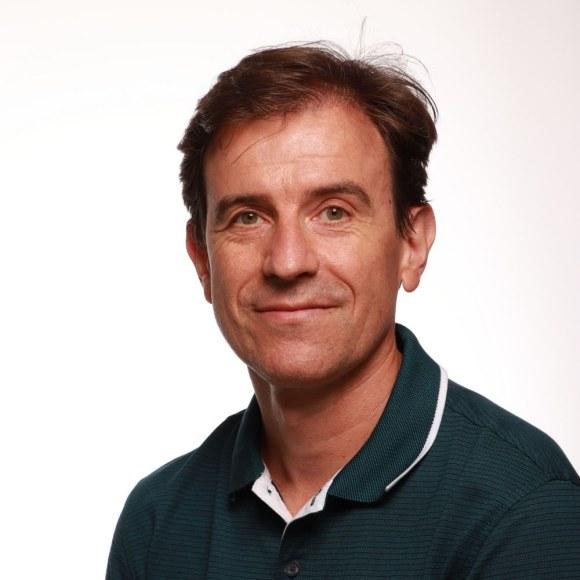
Members
Other members
Baris Ördek
Contact persons
For any inquiries or collaborations please do not hesitate to contact us.