Our research primarily focuses on methodological and empirical research of machine learning, network science, statistical computing, and mathematical modeling, with a special emphasis on time-series modeling and information cascades. In finance, our main area of application, we utilize both contemporary data science techniques and more traditional statistical methods, working with rich data sets.
Research focus and goals
The core objectives are: i) developing Machine Learning methods to analyze and forecast limit order book markets, ii) modelling information cascades within stock markets, iii) applying network inference methods across various domains, including but not limited to finance, iv) modeling stock market volatility, and v) developing option pricing strategies. These aims hold relevance for both the academic sector and industry professionals in financial risk management and market surveillance.
Impact
We have unique, extensive data sets, including investor-level data spanning over 20 years on a daily basis, encompassing more than 1 million Finnish investors. These data sets enable us to analyze and model the actual behavior of individuals in financial decision-making processes. In addition, we employ massive ultra-high-frequency limit order book data from both Nordic and US stock markets.
This vast array of data, coupled with machine learning techniques, enables us to understand how markets are shaped by the information contained in limit order books, a topic of significant importance to traders, risk managers, market makers, and financial regulators. Furthermore, our research in the spreading of insider information within stock markets are of utmost significance, both in academic research and in practical applications.
Leader
Juho Kanniainen
Professor, Computing Sciences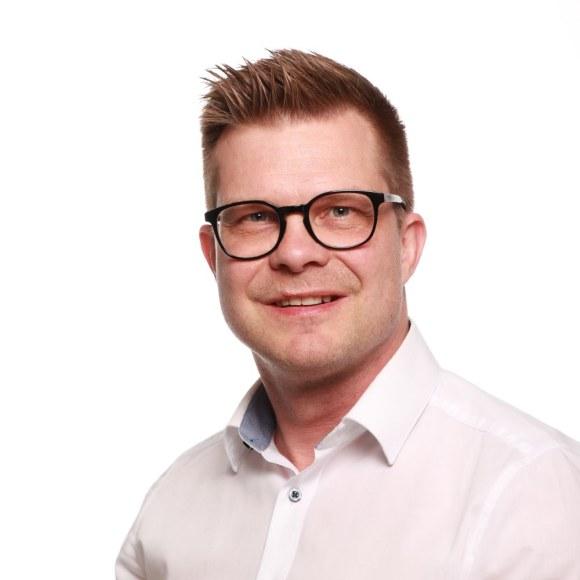