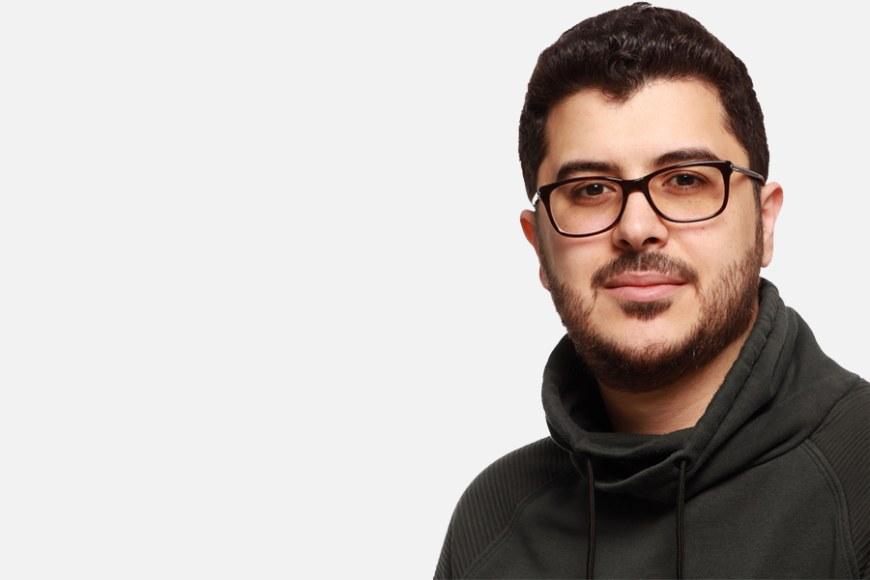
A time-frequency representation, obtained by a time-frequency distribution (TFD), offers access to the signal time-varying spectrum. The Wigner-Ville distribution (WVD) is the most famous quadratic member of Cohen’s class of TFDs and yields optimal representation for mono-component linearly frequency modulated (LFM) signals.
Generally, the WVD suffers from interference patterns named cross-terms which obscure the true information readability and hinder interpretation. The cross-terms severity can be reduced via a smoothing filter which yields high-performing TFDs that are characterized by minimal cross-terms and excellent resolution.
“However, current limitations prevent us from characterizing the TFD performance in terms of accuracy and resolution which hinder objectively comparing different TFDs. As a brain disease that causes seizures, epilepsy can be life-threatening for newborns,” says Al-Sa’d.
The EEG is a standard non-stationary non-invasive measurement that facilitates detecting seizures. However, newborn seizure detectors require large, annotated EEG databases for training, validation and testing.
“The simulated signals or EEG models that are capable of mimicking both healthy (background) and abnormal (seizure) EEG patterns are of great importance for augmentation and further understanding for the EEG dynamics,” he adds.
In his thesis, Mohammad Al-Sa’d investigates these knowledge gaps. He also introduces the PW-WVD as an optimal TFD, proposes new TFD selection strategies, designs a process and derives novel accuracy and resolution measures to evaluate the TFD performance, and offers new feature domains to extract meaningful information from multi-channel EEG recordings. In addition, Al-Sa’d develops a multi-sensor newborn EEG model that takes into account its temporal, spectral, and spatial characteristics, and proposes an adaptive multi-user multiple-modality signal compression paradigm based on deep learning techniques.
In brief, his thesis sheds the light on a fundamental flaw in the design process of computationally expensive TFDs; they do not maximize both the TFD accuracy and resolution. Moreover, the EEG electrical manifestation is confined to some set of electrodes.
“This means that channel selection is paramount and inter-sensor understanding can improve the efficacy of EEG applications,” concludes Mohammad Al-Sa’d.
The doctoral dissertation of Mohammad Al-Sa’d in the field of signal processing titled Time-Frequency Analysis—Application to Electroencephalogram Signal Processing will be publicly examined in the Faculty of Information Technology and Communication Sciences of Tampere University at 12 o’clock on 19 August 2022 at Hervanta Campus in auditorium TB109 of the Tietotalo (Korkeakoulunkatu 1, Tampere). Doctor John O’Toole from University College Cork in Ireland will serve as the Opponent during the thesis defence. The Custos will Professor Moncef Gabbouj from Tampere University.
The dissertation is available online at the https://urn.fi/URN:ISBN:978-952-03-2503-9.