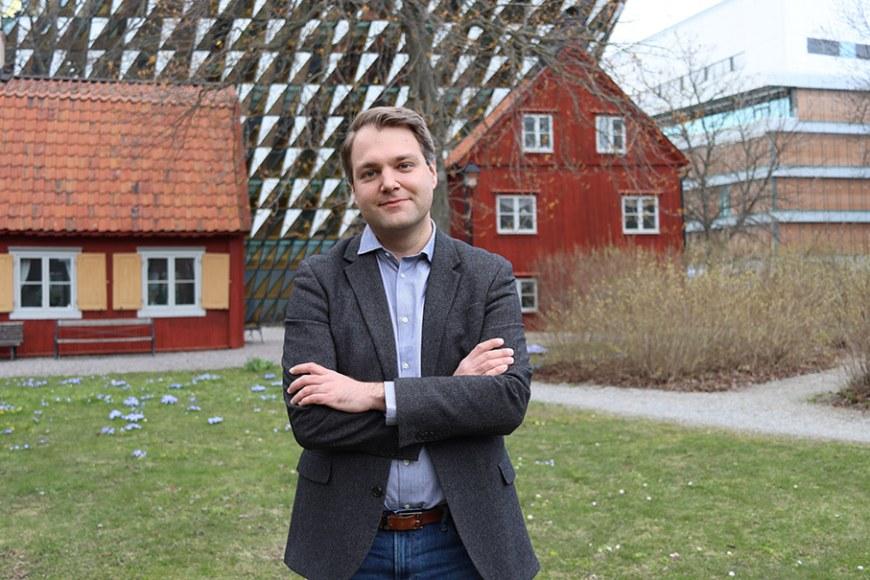
Histopathological assessment consisting in microscopic analysis of tissue specimens is the cornerstone of diagnosing cancer, among other diseases. However, current clinical practice suffers from challenges such as a global shortage of pathologists and considerable inter-pathologist variation in the outcomes of the assessments and the resulting treatment decisions.
Pathology is currently undergoing a transition into a digital discipline, with microscopes being replaced by scanners. Computational analysis of the resulting image data may decrease the workload of pathologists through partial automation and provide decision support for the assessments. In research, digitization of tissue samples also facilitates completely novel, more comprehensive types of analyses.
In the presented doctoral research, machine learning was applied to the detection of cancer cells from digital pathology imaging data. Machine learning is a field of computer science falling under artificial intelligence, which aims at imitating intelligent decision making.
In one of the studies, focusing on breast cancer diagnostics, a machine learning system was trained, using examples provided by pathologists, to discriminate between metastatic breast cancer and normal tissue. The resulting information indicating whether the cancer has spread to the patient’s lymph nodes is critical for treatment planning, but finding the cancer cells is a tedious and time-consuming task for the pathologist.
The most extensive study included in the dissertation presented a system based on deep neural networks, a type of modern machine learning algorithms, which can automatically detect prostate biopsies containing cancer, report the amount of cancer tissue in each sample, and estimate the aggressiveness of the disease using the so called Gleason score.
The study, performed in collaboration with Swedish scientists, utilized more than 8000 digitized biopsies collected as part of the clinical trial STHLM3 to train the system and to evalute its performance.
— This marks the first study globally to demonstrate, based on a large and clinically representative patient cohort, that artificial intelligence can achieve performance comparable to experienced pathologists specialized in urology in diagnosing and Gleason grading prostate cancer, Kimmo Kartasalo says.
The doctoral research additionally focused on 3D histology, the three-dimensional analysis of tissue specimens at microscopical resolution.
Conventionally, tissue sections only represent a limited two-dimensional cross section of the original three-dimensional sample. However, digitization also enables reconstructing the sample in 3D computationally, based on a series of tissue sections. This type of examination can provide a more comprehensive view on biological processes, where the relative positions of different tissue structures and cell types in three-dimensional space are relevant.
— However, the 3D reconstruction is a complex digital image processing problem, and the development of reliable algorithms for the task is further complicated by the difficulty associated with measuring the accuracy of the resulting 3D models.
The presented studies demonstrate means for numerically evaluating the accuracy of 3D reconstructions and report a comparative analysis of various algorithms proposed for the task.
In summary, the doctoral research shows that artificial intelligence achieves expert level performance in the histopathological diagnosis of breast and in particular prostate cancer. The system for assessing prostate biopsies could lower the workload of pathologists, improve patient safety and decrease the uncertainty associated with Gleason grading. The system is currently being further developed in view of clinical adoption. Moreover, a characterisation of the performance of algorithms intended for three-dimensional analysis of tissue samples can facilitate further developing 3D histology from a prototype method into a more broadly adopted tool for biomedical research.
Kartasalo comes originally from Joensuu, Finland, and performed his doctoral research in the bioimage informatics research group at Tampere University.
The doctoral dissertation of M.Sc. (Tech.) Kimmo Kartasalo in the field of biomedical engineering, Machine learning and 3D reconstruction methods for computational pathology, will be publicly examined at the Faculty of Medicine and Health Technology of Tampere University at 12 o’clock on Friday May 21, 2021 in the Jarmo Visakorpi auditorium, Arvo building, Arvo Ylpön katu 34, Tampere. Professor Johan Lundin from Karolinska institutet will be the opponent while Docent Pekka Ruusuvuori from the Faculty of Medicine and Health Technology will act as the custos.
The event can be followed via remote connection.
The dissertation is available online at
http://urn.fi/URN:ISBN:978-952-03-1953-3
Photo: Nita Mulliqi