Genomic biomarkers do not provide any biological understanding of cancer
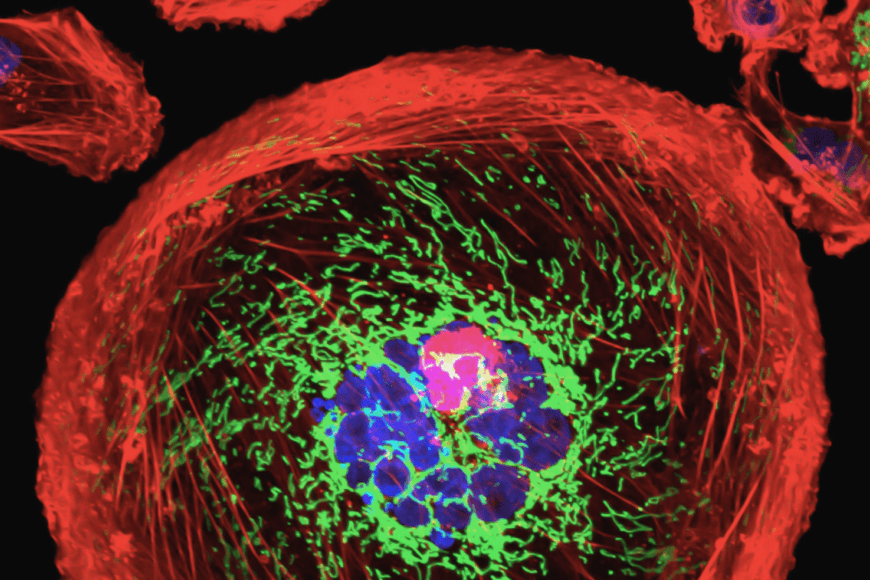
The research group led by Associate Professor Frank Emmert-Streib studies the predictive and biological utility of prognostic biomarkers, i.e., a set of established markers consisting of active genes. They recently conducted large-scale gene expression analyses of 80 established signatures of breast and prostate cancer and discovered that while these biomarkers are successful in predicting cancer outcome, they do not capture any biological meaning.
This result is important because it is generally believed that aside from predicting the progression of patients, such prognostic biomarkers also provide valuable information about disease mechanisms and the underlying molecular processes that cause a disorder. The research group showed by their analyses that this is not the case.
“In our study, we analysed genomic biomarkers of breast and prostate cancer. The problem we found relates to the biological meaning of already established prognostic gene expression signatures. Put simply, prognostic markers do not provide sensible biological information and molecular explanations about the underlying disorder,” Emmert-Streib explains.
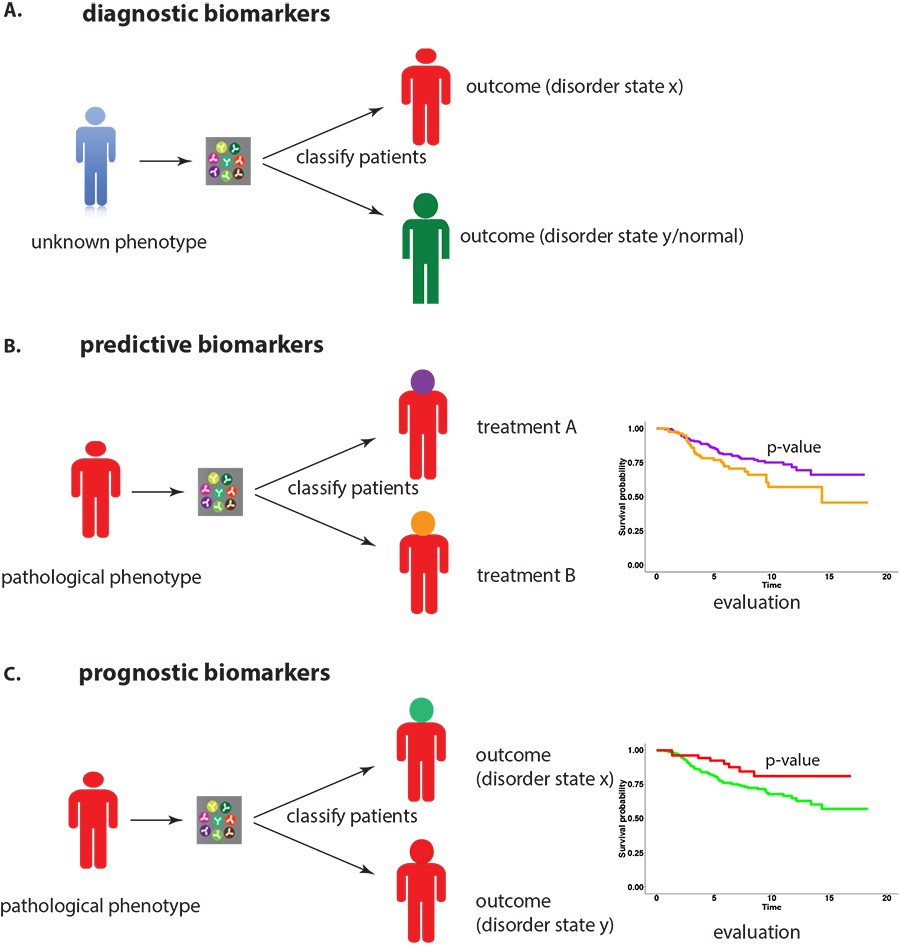
In general, prognostic biomarkers can have an important role in clinical practice because they allow the stratification of patients in terms of predicting the outcome of a disorder. Known obstacles for developing such markers include the lack of robustness when using different data sets and limited concordance among similar signatures. The above-mentioned results provide a new and fundamental problem which could lead to a change in the research paradigm of genome-based research in medicine with important implications for diagnostics and prognostics.
“Our framework can be applied to all biomarker studies based on high-dimensional data. This prevents studies from drawing false conclusions about the biological meaning of signatures,” Emmert-Streib adds.
Are there general limits to the explainability of models?
Conceptually, the above analysis deals with the selection of features from high-dimensional data. From an AI (artificial intelligence) perspective, a biological utility would imply an explainable model which are currently under intense investigation in all areas of science.
According to Frank Emmer-Streib, the investigation of prognostic biomarkers for predicting cancer progression is important beyond cancer.
“First, it provides clarity about the utility of biomarkers/features. Second, it can lead to novel insights regarding the difference of diseases because it can be expected that our results are disorder specific. Third, it may reveal a general property of explainable models,” he says.
The researchers are seeking for an answer to what features make a model explainable. Even though they studied data from biomedical experiments they believe the results are also important for explainable AI models in general.
The article Are There Limits in Explainability of Prognostic Biomarkers? Scrutinizing Biological Utility of Established Signatures was published in MDPI Cancers and Prognostic gene expression signatures of breast cancer are lacking a sensible biological meaning in Nature.
Read Kalifa Manjang’s doctoral dissertation press release on Analysis of prognostic Gene expression Signatures of Breast and Prostate Cancer.
The research is carried out in collaboration with Olli Yli-Harja and Anssi Auvinen from the Faculty of Medicine and Health Technology at Tampere University, Matthias Dehmer from Swiss Distance University of Applied Sciences, Switzerland, Shailesh Tripathi from University of Applied Sciences Upper Austria, Austria, and Galina Glazko from University of Arkansas for Medical Sciences, USA.
Further information
Frank Emmert-Streib
+358 50 301 5353
frank.emmert-streib [at] tuni.fi