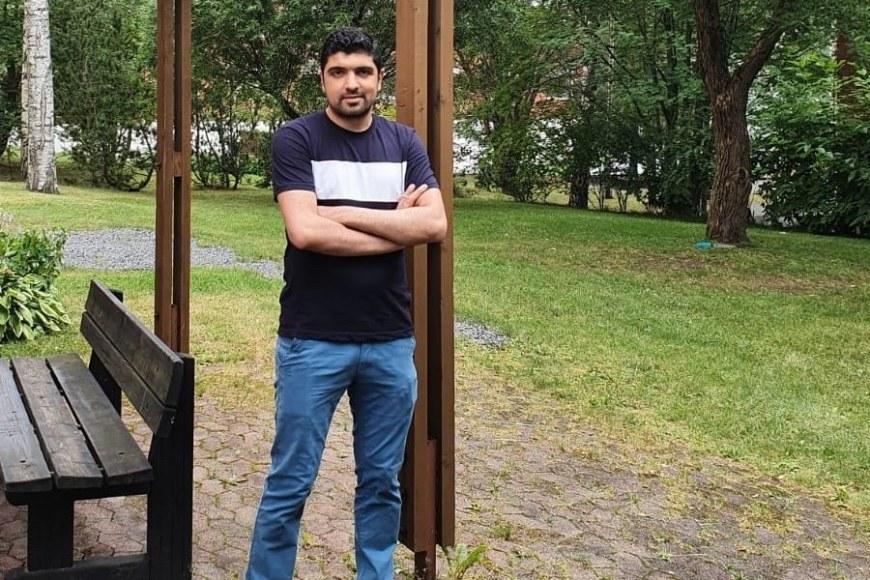
By focusing on the concept of feature diversity within hidden layers, Firas Laakom sheds new light on the inner workings of these powerful learning systems, demonstrating that learning diverse features is crucial to achieve strong generalization. Furthermore, in his dissertation, Firas shows how to leverage this concept of feature diversity, by proposing novel regularization techniques, to boost the performance of neural networks.
In a society increasingly dependent on artificial intelligence, grasping the inner workings of neural network performance is crucial. Firas's doctoral dissertation reveals the significance of diverse feature acquisition, showcasing its link to enhanced generalization abilities through rigorous theoretical examination and practical validation. He explores, why having a mix of different “brain cells” (neurons) in artificial neural networks is crucial to make smart decisions.
“Think of artificial neural networks like a classroom. Just as having diverse students with different perspectives benefits learning, having a mix of different ‘brain cells’ (neurons) in these networks is crucial for making smart decisions. When these neurons in their hidden layers learn to capture different and diverse patterns or features, neural networks thrive.”
Still, why does this matter?
“When everyone thinks the same way, they might find it difficult to solve new problems. This applies to both students in a classroom and the ‘brain cells’ in a neural network. The diversity is the key to making smarter, and more reliable AI systems,” Firas explains.
Not just the theory but also practical solutions
His doctoral dissertation does not just stop at the theory of neural networks features’ diversity but also provides practical solutions. He proposes ways to promote feature diversity in neural networks, helping them perform better on unseen data in real-world tasks such as classification, medical diagnoses, or recommending your next favourite song or film.
"At its core, the research addresses the crucial question about why neural networks perform well in some scenarios and not others," states Firas.
By zooming in on the concept of feature diversity the variety of pattern captured by different neurons within the hidden layers of neural networks the dissertation introduces a novel perspective on generalization.
Neural networks operate in two distinct stages: first, they learn diverse features or representations through intermediate layers, before making final predictions. While the importance of diverse feature learning at the first stage has been empirically observed, this dissertation fills a critical gap by providing rigorous theoretical justification.
Building upon these theoretical insights, the dissertation introduces a new set of data-dependent diversity-inducing regularizers. These novel techniques are designed to encourage the learning of diverse features during training, leading to improved performance across various neural network architectures and tasks.
Firas conducted his doctoral research at SAMI research group at Tampere University. Nowadays, he works as a Researcher in KAUST university under professor Juergen Schmidhuber supervision.
Public defence on Monday 18 March
The doctoral dissertation of MSc (Tech) Firas Laakom in the field of signal processing and machine learning entitled Feature Diversity in Neural Networks: Theory and Algorithms will be publicly examined at the Faculty of Information Technology and Communication Sciences of Tampere University at 12:00 on Monday 18th March 2024. The Opponent will be professor Saikat Chatterjee from KTH - Royal Institute of Technology. The Custos will be professor Moncef Gabbouj from the Faculty of Information Technology and Communication Sciences. The public defence will be held remotely.
The doctoral dissertation is available online
The public defence can be followed via remote connection (Zoom)
Meeting ID: 689 2257 9042
Passcode: 120958