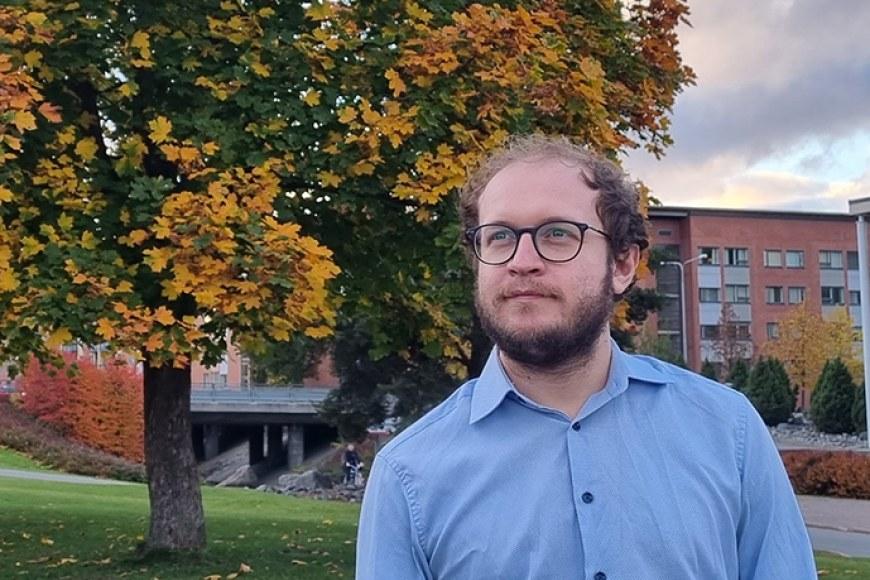
Immersive technologies aim to create virtual worlds for us to experience or aim to extend our perception of reality. All our senses play a role in our perception of reality and arguably vision is the sense in which we rely the most. Perhaps this is one of the reasons why virtual and augmented reality applications which are currently in use primarily appeal to our visual system.
Geometric and visual data obtained by volumetric capture devices are represented as point clouds which are unordered sets of points in 3D space possibly accompanied by attributes such as color.
“In the form of a point cloud, one can store the essential information about a physical object that can be used to recreate a virtual instance of the object for visual experience,” Kaya says.
Data compression seeks ways to represent data in ways that reduce memory usage. Lower memory usage and faster communication of data make a positive impact on our lives by saving resources.
“Reduction in memory usage is made possible by understanding and making use of the redundancy present in the data,” he explains.
Newly introduced data types provide new challenges for data compression. Recently, machine learning approaches became widely popular in several fields of computer science and offered novel efficient ways to represent data. In these approaches, the models explaining redundancy in the data can be learned from the data itself or from similar data.
The doctoral dissertation of MSc (Tech) Emre Can Kaya in the field of computing and electrical engineering titled Visual and Geometric Data Compression for Immersive Technologies will be publicly examined in the Faculty of Information Technology and Communication Sciences (ITC) at Tampere University at 12 o’clock on Friday 4 November 2022. The Opponent will be Assoc. Professor Simone Milani from University of Padova. The Custos will be Professor Ioan Tabus from Tampere University.
The dissertation is available online.
Photo: Andrea Corsini