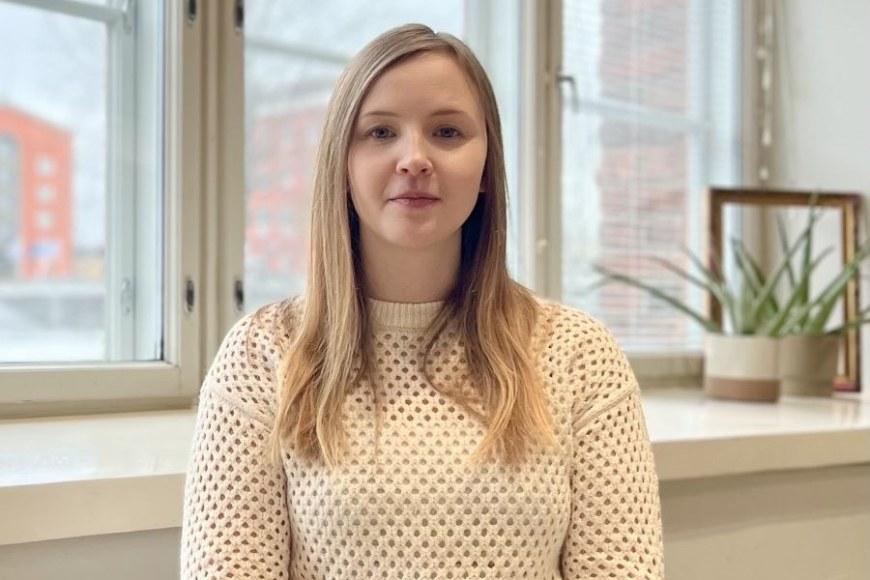
Chronic diseases burden both patients and the healthcare system. Many chronic diseases lead to functional decline as the disease progresses and require frequent follow ups with the doctor. In addition to disease specific symptoms, the patient may experience sleep problems, daytime sleepiness, and fatigue, which decline their quality of life. As the general population ages and obesity increases, the risk of chronic disease increases, and they are expected to become more and more prevalent.
For many uncurable chronic diseases, the symptoms can still be eased, and the progression of the disease can be decelerated. In her doctoral dissertation, MSc (Tech) Emmi Antikainen explored health data applications across different target time spans with the aim to advance disease state monitoring, promote improved patient outcomes, and ease the burden on healthcare providers.
“In the context of chronic diseases, time is key. Observing changes over varying periods of time can cover several meaningful aspects into disease progression”, states Antikainen.
Higher-Quality and More Readily Available Measurement Data Enhance Opportunities for Supporting Healthcare
In her doctoral dissertation, Antikainen investigated algorithms for clinical decision support with application time spans varying from one night to half a year. The applicability of the algorithms to the clinical use cases was evaluated with realistic measurements that correspond to the real-life use cases.
In the first application, the variations in respiration and heart rate were measured using contact-free radar technology. Such near real-time vital sign monitoring may promptly capture changes in patient state, e.g. that of sleep apnea patients. In addition to nocturnal monitoring, similar technology could be applied at nursing homes. The study results indicated that conventional algorithms based on high frequency input can be well suited to meet the requirements for clinical algorithms.
The aim of the second use case was to find links between subjective experiences associated with chronic diseases, such as fatigue and daytime sleepiness, and biosignals collected with a wearable sensor while the participants went on with their daily lives. Objective measurements of subjective experiences could advance the discovery of personalized care solutions and promote new research studying the effects that treatments and interventions may have on the quality of life. In the study, the free-living measurements were found more valuable when the context could be automatically detected.
In the final use case, the six-month mortality risk among cardiac patients was predicted from electronic health records. The longer time span in predictive algorithms may provide a better chance of finding a life-saving solution for a patient or assist weighing the risks and benefits of high-risk operations. This study applied the same technology that the famous ChatGPT is based on.
“Modern technology has enabled the mining of bigger and bigger data, and more efficient analytics. Thus, the exploration of long-term data has also become easier. The benefits of artificial intelligence algorithms were specifically noted in the context of long-term but episodic health records. Simultaneously, however, the research showed that they may not be applicable for applications covering a wide range of patients even if the diseases were physiologically related”, says Antikainen.
Antikainen conducted her doctoral research at the VTT Technical Research Centre of Finland Ltd. Nowadays she works as a data scientist at Oura Health Oy, the smart ring company.
Public defence on Friday 15 March
The doctoral dissertation of MSc (Tech) Emmi Antikainen in the field of signal processing and machine learning titled Time series analytics for decision support in chronic diseases will be publicly examined at the Faculty of Information Technology and Communication Sciences of Tampere University in room TB109 of the Tietotalo building (address: Korkeakoulunkatu 1, Tampere) at 12:00 on Friday 15 March 2024. The Opponent will be professor Tapio Seppänen from the University of Oulu, Finland. The Custos will be professor Moncef Gabbouj from the Faculty of Information Technology and Communication Sciences, Tampere University.